AI Dangers: Loss of Control and Bias
Updated Oct 30, 2023
One of the most pressing dangers of AI is the loss of meaningful control over these systems [1]. As AI becomes more capable and is integrated into societal infrastructure, the implications of losing control over these systems become more concerning. AI systems are less reliant on explicit, easily specified rules and more reliant on complex algorithms that can be difficult to understand and control.
Another danger of AI is the potential to perpetuate and even amplify biases [2]. Many AI systems are trained on data sets that reflect the biases and prejudices of the people who created them. This can lead to discrimination and unfair treatment of certain groups.
AI Dangers: Job Automation and Security Concerns
AI-powered job automation is a pressing concern as the technology is adopted in marketing, manufacturing, and healthcare [3]. Eighty-five million jobs will be lost to automation between 2020 and 2025, with Black and Latino employees especially vulnerable.
AI systems can significantly threaten security and privacy [5]. As AI becomes more integrated into our daily lives, it will have access to vast amounts of personal data. This data can be vulnerable to hacking, theft, and misuse.
AI Dangers: Autonomy and Unintended Consequences
As AI systems become more autonomous, there is a risk that they will act in ways that are unpredictable and even dangerous [1]. This can lead to accidents and incidents that are difficult to prevent or mitigate.
AI systems can have unintended consequences that are difficult to predict [4]. As AI becomes more integrated into our society and economy, it will significantly impact many aspects of our lives. These impacts may be difficult to anticipate and prepare for.
Navigating AI Dangers: A Path Forward
AI can potentially transform many industries and improve our lives in many ways. However, it is important to recognize this technology’s significant risks and dangers. The hazards of AI include loss of control, bias, job automation, security and privacy risks, autonomy, and unintended consequences. As AI continues to advance, it is important to take these risks seriously and work to mitigate them. By doing so, we can ensure that AI is used to benefit society.
Loss of Control
One significant danger of AI is losing control over the technology. As AI systems become more complex and autonomous, it may become difficult for humans to fully understand and predict their behaviour. This could lead to systems making decisions humans do not intend or want. Ensuring proper oversight, governance, and safety measures can help maintain human control over AI.
The potential loss of control over AI systems is a significant concern. As AI technology advances and becomes more complex and autonomous, there is a risk that humans may struggle to fully understand and predict their behavior. This can lead to AI systems making decisions that humans did not intend or desire, which could have serious consequences. It is crucial to establish proper oversight, governance, and safety measures to address this issue. Here are some key points to consider:
1. Explainability and Transparency: Efforts should be made to enhance the explainability and transparency of AI systems. Researchers and developers should strive to design AI models and algorithms that clearly explain their decision-making processes. This can help humans understand and trust the system’s behavior.
2. Ethical Frameworks: It is essential to develop and adhere to ethical frameworks in the development and deployment of AI. These frameworks should address issues such as fairness, accountability, and transparency. They can guide the behaviour of AI systems and ensure that they align with human values and intentions.
3. Regulatory Policies: Governments and regulatory bodies are critical in establishing policies and regulations for AI development and deployment. These policies should address safety, privacy, and ethical concerns while promoting innovation. Striking the right balance ensures that AI technology is developed and used responsibly.
4. Continuous Monitoring and Auditing: AI systems should be subject to ongoing monitoring and auditing to ensure their compliance with safety and ethical standards. Regular assessments can help identify and mitigate potential risks and biases in the system’s decision-making processes.
5. Human-in-the-loop Approach: Adopting a “human-in-the-loop” approach can maintain human control over AI systems. This involves involving humans in the decision-making process and incorporating their expertise and judgment. Humans can review and validate the decisions made by AI systems, providing an additional layer of oversight.
6. International Collaboration: The development of global standards and collaboration between countries can facilitate consistent regulation and oversight of AI technology. It can help prevent a fragmented approach and ensure that safety measures and governance practices are universally adopted.
By implementing these measures, we can strive to maintain human control over AI technology and address the potential risks associated with its increasing complexity and autonomy. It is crucial to approach AI development and deployment with responsibility, ethics, and a focus on long-term societal benefits.
Bias
AI systems are only as good as the data used to train them. Since data often reflects human biases, AI models can easily inherit and amplify biases related to race, gender, and other attributes. Auditing data and models for bias and training systems on a diverse range of data can help reduce unfair discrimination by AI.
Bias in AI systems is a significant concern. AI models learn from the data they are trained on, and if that data contains biases, the models can perpetuate and amplify those biases. This can lead to unfair discrimination and perpetuation of societal biases. Here are some key points to consider in addressing bias in AI systems:
1. Data Auditing: It is crucial to conduct thorough audits of the data used to train AI systems. This involves identifying potential biases and evaluating the representativeness and diversity of the data. Data auditing can help uncover and understand biases present in the training data, allowing for corrective measures to be taken.
2. Diverse Training Data: Training AI systems on a diverse range of data can help mitigate biases. By incorporating data from different sources and perspectives, AI models can have a broader understanding of the world and reduce the risk of perpetuating biased outcomes.
3. Bias Mitigation Techniques: Various techniques can be employed to mitigate bias in AI systems. For example, pre-processing techniques such as data augmentation and balancing can help address imbalances in the training data. Algorithmic techniques, such as fairness-aware learning and debiasing methods, can reduce bias in decision-making processes.
4. Multidisciplinary Teams: Building diverse and multidisciplinary teams can help identify and address biases in AI systems. By bringing together individuals from different backgrounds, including ethicists, social scientists, and domain experts, a more comprehensive examination of biases can be undertaken, leading to more robust and fair AI systems.
5. Continuous Monitoring and Evaluation: AI systems should continuously monitor and evaluate bias, even after deployment. This involves ongoing evaluation of the system’s performance and impact on user groups. Regular assessments can help identify and address any emerging biases or unintended consequences.
6. Ethical and Regulatory Guidelines: Ethical guidelines and regulatory frameworks can play a crucial role in addressing bias in AI. These guidelines should emphasize the importance of fairness, non-discrimination, and transparency in AI development and deployment. They can provide a foundation for developers, organizations, and policymakers to ensure that AI systems are developed and used responsibly and unbiasedly.
Addressing bias in AI systems requires a comprehensive and proactive approach. By auditing data, training on diverse datasets, employing bias mitigation techniques, fostering various teams, monitoring performance, and adhering to ethical and regulatory guidelines, we can work towards reducing unfair discrimination and promoting the development of more equitable and unbiased AI systems.
Job Automation
AI, especially machine learning and robotics, has the potential to automate many jobs currently performed by humans. While this may increase productivity and efficiency, it also risks displacing many workers. Preparing for this disruption through education, retraining programs, and social safety nets can help workers and communities transition to a future with AI.
AI, particularly machine learning and robotics, has the potential to automate a wide range of jobs currently performed by humans. This automation can increase productivity and efficiency, but it also raises concerns about job displacement and the need to support workers during this transition. Here are some key considerations:
1. Education and Skills Development: To prepare for the disruption caused by job automation, there is a need to focus on education and skills development. Promoting STEM education (science, technology, engineering, and mathematics) and emphasizing skills that complement AI, such as critical thinking, creativity, and problem-solving, can help individuals adapt to the changing job market.
2. Retraining and Reskilling Programs: Implementing retraining and reskilling programs is crucial to enable workers to acquire new skills and transition into emerging roles. These programs should be designed in collaboration with industry stakeholders and educational institutions to ensure they align with the evolving demands of the job market.
3. Lifelong Learning: The rapid advancement of AI and technology necessitates a shift towards lifelong learning. Encouraging a culture of continuous learning and providing accessible avenues for upskilling and reskilling throughout one’s career can help individuals stay relevant and adaptable in the face of automation.
4. Job Creation and Entrepreneurship: While some jobs may be automated, new job opportunities can also emerge due to AI. Encouraging entrepreneurship and fostering innovation can help create new ventures and industries, thereby generating employment opportunities. Governments and organizations can support startups and provide resources for individuals to pursue entrepreneurial endeavours.
5. Social Safety Nets: Displaced workers may face challenges during transition. Establishing comprehensive social safety nets, such as unemployment benefits, job placement services, and income support programs, can help mitigate the adverse effects of job displacement and provide a safety net for affected individuals.
6. Collaboration between Stakeholders: Addressing the impact of job automation requires collaboration between various stakeholders, including governments, businesses, educational institutions, and labour organizations. By working together, these stakeholders can develop comprehensive strategies to navigate the transition and ensure the well-being of workers and communities.
Security and Privacy Risks
As AI is integrated into critical systems like transportation, healthcare, and infrastructure, it also exposes those systems to new security threats and vulnerabilities. Malicious actors could exploit AI to cause harm, steal data, or disrupt operations. Ensuring the security and privacy of AI systems should be a top priority for developers, regulators, and users.
Integrating AI into critical systems introduces new security and privacy risks that must be addressed. Here are some key considerations regarding safety and privacy in AI systems:
1. Threats and Vulnerabilities: AI systems can become targets for malicious actors who may seek to exploit vulnerabilities or manipulate the system for their own gain. Developers must be mindful of potential threats such as data poisoning, adversarial attacks, and model inversion attacks. Conducting rigorous security assessments and testing can help identify and mitigate these vulnerabilities.
2. Robust Data Protection: AI systems heavily rely on data, and protecting the privacy and security of that data is crucial. Implementing robust data protection measures, such as encryption, access controls, and secure data storage, can help safeguard sensitive information and prevent unauthorized access or data breaches.
3. Adversarial Attacks and Defense: Adversarial attacks involve manipulating AI systems by feeding them maliciously crafted input data. Developing robust AI models against adversarial attacks and investing in techniques such as negative training can help enhance system defences and make them more resilient to such attacks.
4. Secure Development Practices: Incorporating secure development practices throughout the AI system’s lifecycle is essential. This includes certain coding practices, regular security updates and patches, and adherence to established security standards and protocols. Security audits and vulnerability assessments can also help identify and address potential security weaknesses.
5. Regulatory Frameworks and Standards: Governments and regulatory bodies play a crucial role in establishing frameworks and standards for AI security and privacy. These regulations can set requirements for security measures, data protection, and privacy safeguards. Compliance with these regulations helps ensure that AI systems are developed and deployed in a secure and privacy-respecting manner.
6. User Awareness and Education: Users of AI systems, whether individuals or organizations, should be educated about the security and privacy risks associated with AI technology. Promoting awareness of best practices, such as strong authentication, regular software updates, and safe data handling, can empower users to protect themselves and their systems proactively.
7. Collaboration and Information Sharing: Collaboration and information sharing among stakeholders, including developers, researchers, and security experts, are vital in addressing security and privacy risks. Sharing knowledge about emerging threats, vulnerabilities, and best practices can help the AI community avoid potential risks and collectively work towards more secure and privacy-preserving AI systems.
Ensuring the security and privacy of AI systems is an ongoing and evolving process. By adopting a proactive and multi-faceted approach that includes secure development practices, robust data protection, compliance with regulations, and user education, we can mitigate security risks and safeguard the integrity and privacy of AI systems as they become increasingly integrated into critical domains.
Surging Enthusiasm for AI: Few Stocks Drive the Market
The excitement surrounding AI is rapidly growing, signalling the start of a new bull market, according to many. However, it’s important to note that only a few stocks, less than 9, fuel the Nasdaq and SP500. Despite AI being hailed as the next saviour, a crucial aspect is overlooked by those making these claims. The masses are typically drawn to simple concepts, following the principle of KISS (keep it simple, stupid). Unfortunately, current AI bots do not align with this principle.
Simply put, people desire an effortlessly understanding and user-friendly AI personal assistant. However, existing chatbots often provide inadequate responses, requiring repeated prompts. Sometimes, these chatbots can deliver impressive answers with the right prompts, but that’s where the problem lies. Who wants to spend time learning how to communicate with a prompt effectively? It’s like going back in time to the era of DOS prompts.
A recent study revealed that only 20% of individuals actively utilize these models, and even among them, it’s likely that less than 5% truly comprehend their usage. As a result, the significant investments made by major companies in a flawed product seem unwise. The flaw originates from a straightforward reason: AI cannot yet consider human fallibility. In simpler terms, it struggles to engage effectively with individuals not well-versed in complex technologies. Until these changes, the general public will not fully embrace AI.
Conclusion on AI Dangers
You raise some valid concerns about the dangers of AI. Here are some key points I’d like to highlight:
• Biases: Many AI systems inherit and amplify human biases, which can lead to unfair discrimination against certain groups. Auditing for bias and training on diverse data can help mitigate this.
• Job automation: AI has the potential to automate many jobs, especially those held by vulnerable groups. We need plans to help workers transition and adjust.
• Security: As AI becomes more integrated into our lives, it exposes critical systems to new security threats. Developers must prioritize securing AI systems.
• Autonomy: As AI gains more autonomy, there is a risk it may act in unintended or dangerous ways. Proper oversight and safety measures are needed to maintain human control.
• Usability: Most people struggle to use existing AI systems effectively. Companies must focus on creating more intuitive and user-friendly AI that people can easily adopt.
In summary, while AI offers benefits, we must also be aware of its dangers – especially biases, threats to jobs and security, and the need for proper governance and oversight. We can maximize AI’s benefits while mitigating its risks with care and foresight.
Read, Learn, Grow: Other Engaging Articles You Shouldn’t Miss
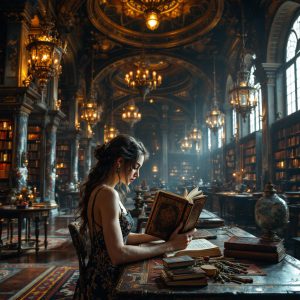
Market Psychology Books: Real Life, Not Books, Wins the Game
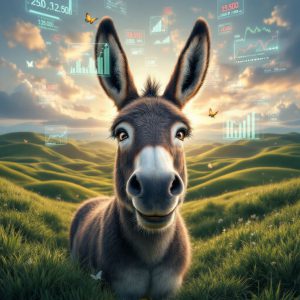
Buy When Others Are Fearful, Or Lose Like a Fool
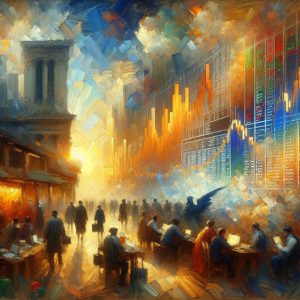
Analyzing Trends: Stock Market Forecast for the Next 6 Months
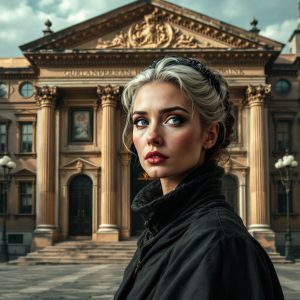
The Dow Theory: Still Relevant or Outdated? How to Improve It
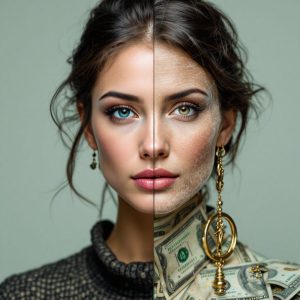
The Virtue of Selfishness: Unlock a Better Life and Success
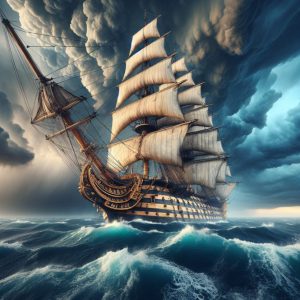
Stock Market Forecast for Next 3 Months: Trends to Watch, Predictions to Ignore
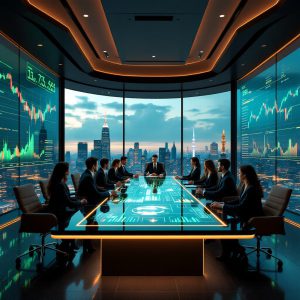
Examples of Groupthink: Instances of Collective Decision-Making
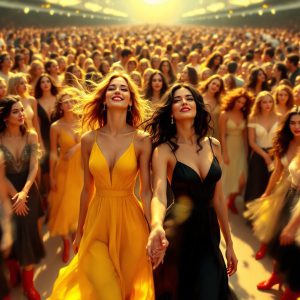
Collective Sentiment: Mass Mindset Blocks Financial Success
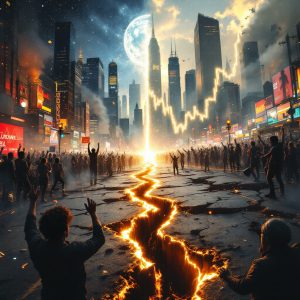
Stock Market Crash Coming 2025: Genuine Risk or Overblown Fear
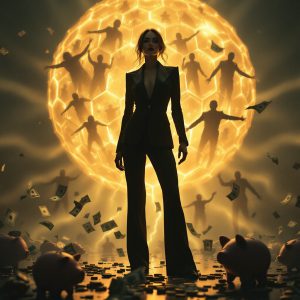
The Lemming Effect Enigma: Unraveling the Hive Mind
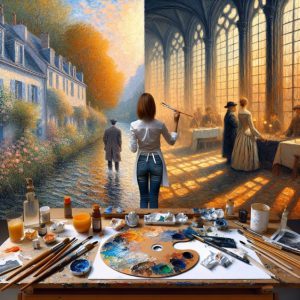
Dow theory Forecasts: Alternative could be better
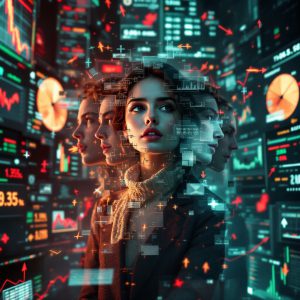
Perception Manipulation: Mastering the Market with Strategic Insight
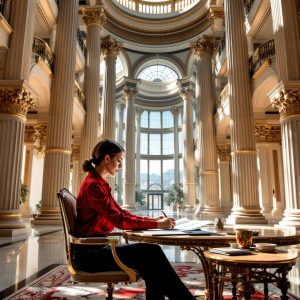
Why is Investing a More Powerful Tool to Build Long-term Wealth Than Saving
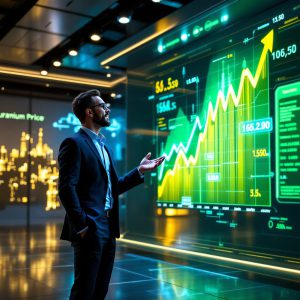
Dow Utilities And The Tactical Investor Dow Theory
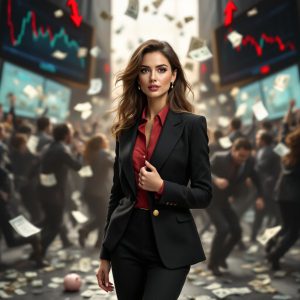